Giving Design Jobs to Machines
Giving Design Jobs to Machines
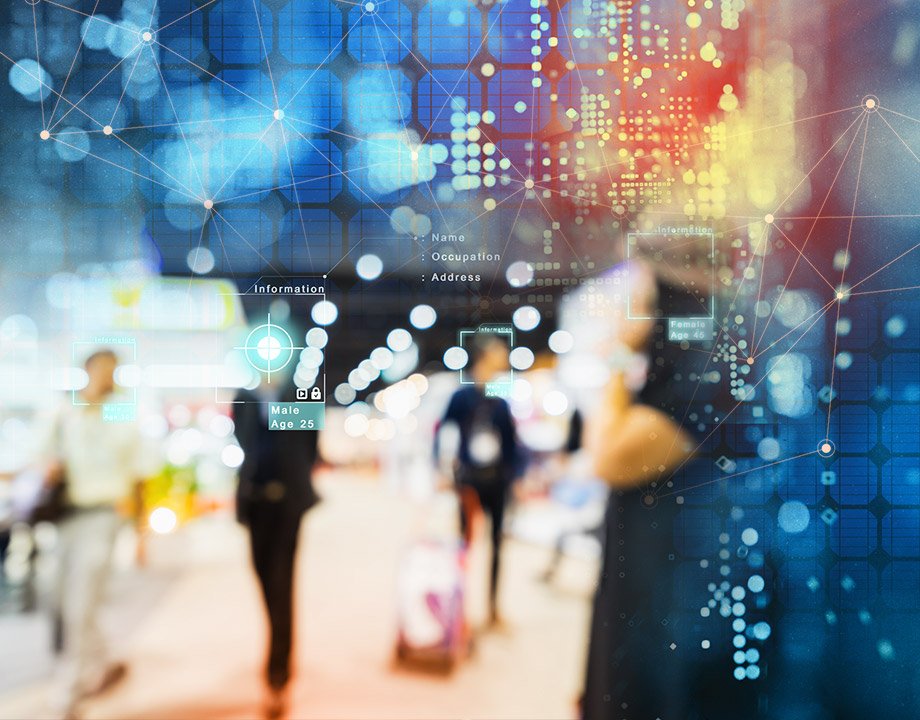
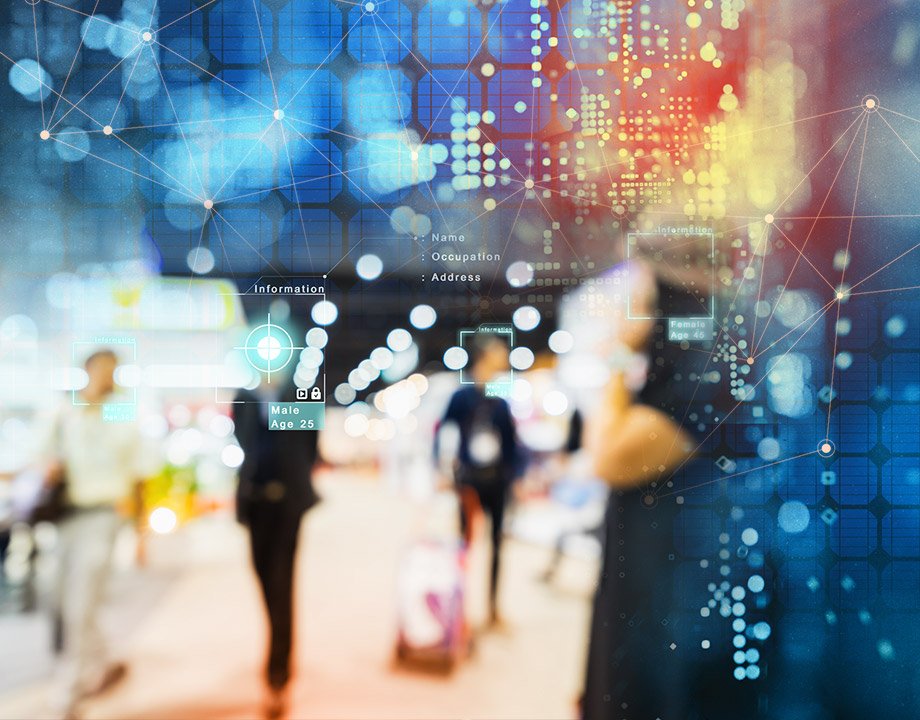
Researchers at several universities are pushing the frontiers of machine learning into the realms of engineering design.
For consumers, machine learning largely takes place behind the scenes, by helping Amazon know exactly what type of books you like to read, for example. Over time, algorithms learn, and apply, your preferences.
Now researchers at several universities are pushing the frontiers of machine learning into the realms of engineering design. To begin, several academic labs are looking at ways to use machine learning for better solar-cell design.
“We’re looking at a non-traditional way of doing machine learning,” said Soumik Sarkar, an associate professor of mechanical engineering at Iowa State University in Ames. “Machine learning has been used to make your next Netflix recommendation. The new frontier is trying to see if machine learning can help engineers or scientists do engineering and science better.”
Machine learning is a data-driven approach to problem solving. It uses calculation tools to learn and identify patterns or relationships; to predict results; or to make decisions resulting from the minimal or maximum chance of either error or success, depending on the problem, said Kuan Sun, a professor in the school of energy and power at Chongqing University in China. Researchers there are also looking at machine learning to optimize design and are also, like at Iowa State, beginning with the design of solar cells.
More for You: The Future of Design
The Iowa State group seeks to use machine learning to speed up the engineering design process, said Baskar Ganapathysubramanian, a professor of engineering there who leads the group’s effort. His team’s first goal is to develop machine-learning software that can rapidly identify the types of organic thin film structures that boost solar cell performance and that are easy to manufacture. The broader goal is to demonstrate that machine learning can help rapidly design all kinds of technologies and engineered parts and assemblies.
The method the Iowa State researchers is developing begins with already existing knowledge, Ganapathysubramanian said.
“The question is, can I circumvent the need for expensive data collection by using knowledge that the domain already has?” he asked.
If he can, the machine-learning approach to engineering design would save time and money as compared to the traditional design process.
It could return answers more quickly than the prototyping, analysis, and redesign process engineers most often use to optimize their designs, Ganapathysubramanian added.
Sarkar gives the example of an engineer charged with designing a car that can travel 60 miles on one gallon of gas.
“Instead of testing better aerodynamics here, lighter materials there, new engine technology under the hood, the new process would use machine learning to analyze existing data as well as new computer models to come up with an initial design capable of hitting 60 miles per gallon,” Sarkar said.
The machine learning algorithms would scan existing data, learn patterns, make predictions, and help them quickly find the optimal design, he added.
Data-Driven Approach
At Chongqing University, researchers are focusing their machine learning efforts on improving solar-cell design because they believe many improvements can be made to boast the cell’s efficiencies, Sun said.
Editor's Pick: Getting More Energy from Solar Panels
According to the U.S. Department of Energy, photovoltaic cells can convert around 20 percent of the sun’s energy into electricity. Developing new designs could create more efficient, durable, and affordable cells and allow them to become part of more homes, buildings, and other structures.
Sun’s team focuses on organic solar cells, which use organic polymers or molecules to capture the sun’s energy and convert it to electricity. The development of organic solar cells has been slow because it involves the design and synthesis of stable photovoltaic materials, the characterization of photoelectric properties of materials, and the assembly and optimization of photovoltaic cells. This traditional method includes fine control and optimization of chemical synthesis and device preparation, he said.
From the time the organic solar cell was first reported in 1973, there have been fewer than 2,000 kinds of materials synthesized and tested in photovoltaic devices. The experimental data generated in the past half century is valuable, Sun said. But the potential value of the data has not been fully used in the search for high-performance organic photovoltaic materials, he added.
“In order to extract useful information from these data, we need a program that can scan a large number of data sets and extract the relationship between features,” Sun said.
Reader's Choice: Using AI to Manage the Grid
That’s where his team’s data-driven approach will be valuable. The researchers began creating a machine-learning algorithm by assembling a dataset of more than 1,700 potential materials that could be used. They called upon past publications and other literature for this, Sun said.
The machine-learning approach can predict a range of material properties without the need for an in-depth understanding of the chemical or physical principles behind them, he added.
In this way, scientists will be able to test hundreds or thousands of combinations before creating a physical prototype, he said.
While better solar cells are a good thing, Ganapathysubramanian said, it’s not the biggest result that could come from these machine-learning projects.
“The most important outcomes are going to be theory and software tools that allow us to design new technologies in a fast and agile manner,” he said.
Jean Thilmany is a science and technology writer based in St. Paul, Minn.
Now researchers at several universities are pushing the frontiers of machine learning into the realms of engineering design. To begin, several academic labs are looking at ways to use machine learning for better solar-cell design.
“We’re looking at a non-traditional way of doing machine learning,” said Soumik Sarkar, an associate professor of mechanical engineering at Iowa State University in Ames. “Machine learning has been used to make your next Netflix recommendation. The new frontier is trying to see if machine learning can help engineers or scientists do engineering and science better.”
Machine learning is a data-driven approach to problem solving. It uses calculation tools to learn and identify patterns or relationships; to predict results; or to make decisions resulting from the minimal or maximum chance of either error or success, depending on the problem, said Kuan Sun, a professor in the school of energy and power at Chongqing University in China. Researchers there are also looking at machine learning to optimize design and are also, like at Iowa State, beginning with the design of solar cells.
More for You: The Future of Design
The Iowa State group seeks to use machine learning to speed up the engineering design process, said Baskar Ganapathysubramanian, a professor of engineering there who leads the group’s effort. His team’s first goal is to develop machine-learning software that can rapidly identify the types of organic thin film structures that boost solar cell performance and that are easy to manufacture. The broader goal is to demonstrate that machine learning can help rapidly design all kinds of technologies and engineered parts and assemblies.
The method the Iowa State researchers is developing begins with already existing knowledge, Ganapathysubramanian said.
“The question is, can I circumvent the need for expensive data collection by using knowledge that the domain already has?” he asked.
If he can, the machine-learning approach to engineering design would save time and money as compared to the traditional design process.
It could return answers more quickly than the prototyping, analysis, and redesign process engineers most often use to optimize their designs, Ganapathysubramanian added.
Sarkar gives the example of an engineer charged with designing a car that can travel 60 miles on one gallon of gas.
“Instead of testing better aerodynamics here, lighter materials there, new engine technology under the hood, the new process would use machine learning to analyze existing data as well as new computer models to come up with an initial design capable of hitting 60 miles per gallon,” Sarkar said.
The machine learning algorithms would scan existing data, learn patterns, make predictions, and help them quickly find the optimal design, he added.
Data-Driven Approach
At Chongqing University, researchers are focusing their machine learning efforts on improving solar-cell design because they believe many improvements can be made to boast the cell’s efficiencies, Sun said.
Editor's Pick: Getting More Energy from Solar Panels
According to the U.S. Department of Energy, photovoltaic cells can convert around 20 percent of the sun’s energy into electricity. Developing new designs could create more efficient, durable, and affordable cells and allow them to become part of more homes, buildings, and other structures.
Sun’s team focuses on organic solar cells, which use organic polymers or molecules to capture the sun’s energy and convert it to electricity. The development of organic solar cells has been slow because it involves the design and synthesis of stable photovoltaic materials, the characterization of photoelectric properties of materials, and the assembly and optimization of photovoltaic cells. This traditional method includes fine control and optimization of chemical synthesis and device preparation, he said.
From the time the organic solar cell was first reported in 1973, there have been fewer than 2,000 kinds of materials synthesized and tested in photovoltaic devices. The experimental data generated in the past half century is valuable, Sun said. But the potential value of the data has not been fully used in the search for high-performance organic photovoltaic materials, he added.
“In order to extract useful information from these data, we need a program that can scan a large number of data sets and extract the relationship between features,” Sun said.
Reader's Choice: Using AI to Manage the Grid
That’s where his team’s data-driven approach will be valuable. The researchers began creating a machine-learning algorithm by assembling a dataset of more than 1,700 potential materials that could be used. They called upon past publications and other literature for this, Sun said.
The machine-learning approach can predict a range of material properties without the need for an in-depth understanding of the chemical or physical principles behind them, he added.
In this way, scientists will be able to test hundreds or thousands of combinations before creating a physical prototype, he said.
While better solar cells are a good thing, Ganapathysubramanian said, it’s not the biggest result that could come from these machine-learning projects.
“The most important outcomes are going to be theory and software tools that allow us to design new technologies in a fast and agile manner,” he said.
Jean Thilmany is a science and technology writer based in St. Paul, Minn.