Special Journal Issue on Data-Driven Mechanics and Digital Twins for Ocean Engineering
Special Journal Issue on Data-Driven Mechanics and Digital Twins for Ocean Engineering
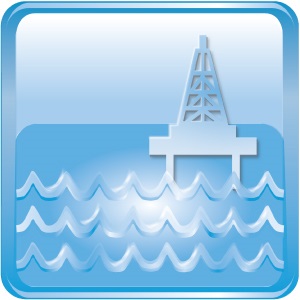
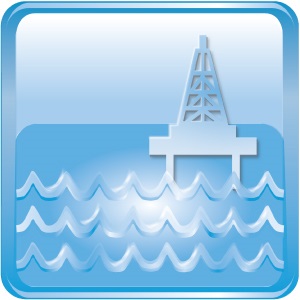
The ASME Journal of Offshore Mechanics and Arctic Engineering is currently accepting manuscripts for a special issue focusing on the topic “Data-Driven Mechanics and Digital Twins for Ocean Engineering.” Authors who are interested in having their manuscripts included in the special issue, to be published in December 2022, should submit their manuscripts by May 31, 2022.
Digital twin technology has emerged as a revolutionary concept for next-generation marine vehicles and offshore/arctic systems. In recent years, advancements in machine learning (ML) methods, sensor technology, the Internet of Things, cloud computing, data acquisition and transmission of massive data along with superior computing power have propelled the development of digital twin technology. Aligning with these emerging trends, one of the key aims of this special issue is the symbiosis of traditional measurements and modeling methods (e.g., computational fluid dynamics and finite element analysis) with data-driven artificial intelligence (AI) and ML techniques for reliable and efficient dynamical predictions (e.g., fluid-structure interaction) in large-scale parametric analysis, uncertainty quantification, optimization, and control.
The special issue seeks to compile recent advances in data-driven techniques and AI/ML techniques in offshore mechanics and arctic engineering, and showcase the application of these methods in a variety of challenging problems related to digital twin technology.
Manuscripts to be included in the special issue of greatest interest include, but are not limited to, the following areas: mechanistic machine learning; reduced-order modeling; digital twins; fluid-structure interaction; computational fluid dynamics; finite element analysis; optimization and control; and uncertainty quantification.
Manuscripts should be submitted electronically to the journal by May 31, 2022, via Journals Connect at journaltool.asme.org. Authors who have an account should log in and select “Submit Paper” at the bottom of the page. Authors without an account should select “Submissions” and follow the steps. At the Paper Submittal page, authors should select “ASME Journal of Offshore Mechanics and Arctic Engineering” and then select the special issue “Data-Driven Mechanics and Digital Twins for Ocean Engineering.” Papers received after the deadline or papers not selected for inclusion in the special issue may be accepted for publication in a regular issue.
The guest editor for the special issue is Rajeev Jaiman, The University of British Columbia, Canada, rjaiman@mech.ubc.ca.
For more information on the ASME Journal of Offshore Mechanics and Arctic Engineering, visit https://asmedigitalcollection.asme.org/offshoremechanics. To learn more about the ASME Journal Program, visit www.asme.org/publications-submissions/journals/information-for-authors.
Digital twin technology has emerged as a revolutionary concept for next-generation marine vehicles and offshore/arctic systems. In recent years, advancements in machine learning (ML) methods, sensor technology, the Internet of Things, cloud computing, data acquisition and transmission of massive data along with superior computing power have propelled the development of digital twin technology. Aligning with these emerging trends, one of the key aims of this special issue is the symbiosis of traditional measurements and modeling methods (e.g., computational fluid dynamics and finite element analysis) with data-driven artificial intelligence (AI) and ML techniques for reliable and efficient dynamical predictions (e.g., fluid-structure interaction) in large-scale parametric analysis, uncertainty quantification, optimization, and control.
The special issue seeks to compile recent advances in data-driven techniques and AI/ML techniques in offshore mechanics and arctic engineering, and showcase the application of these methods in a variety of challenging problems related to digital twin technology.
Manuscripts to be included in the special issue of greatest interest include, but are not limited to, the following areas: mechanistic machine learning; reduced-order modeling; digital twins; fluid-structure interaction; computational fluid dynamics; finite element analysis; optimization and control; and uncertainty quantification.
Manuscripts should be submitted electronically to the journal by May 31, 2022, via Journals Connect at journaltool.asme.org. Authors who have an account should log in and select “Submit Paper” at the bottom of the page. Authors without an account should select “Submissions” and follow the steps. At the Paper Submittal page, authors should select “ASME Journal of Offshore Mechanics and Arctic Engineering” and then select the special issue “Data-Driven Mechanics and Digital Twins for Ocean Engineering.” Papers received after the deadline or papers not selected for inclusion in the special issue may be accepted for publication in a regular issue.
The guest editor for the special issue is Rajeev Jaiman, The University of British Columbia, Canada, rjaiman@mech.ubc.ca.
For more information on the ASME Journal of Offshore Mechanics and Arctic Engineering, visit https://asmedigitalcollection.asme.org/offshoremechanics. To learn more about the ASME Journal Program, visit www.asme.org/publications-submissions/journals/information-for-authors.