AI and Optimization: A Meeting of the Minds
AI and Optimization: A Meeting of the Minds
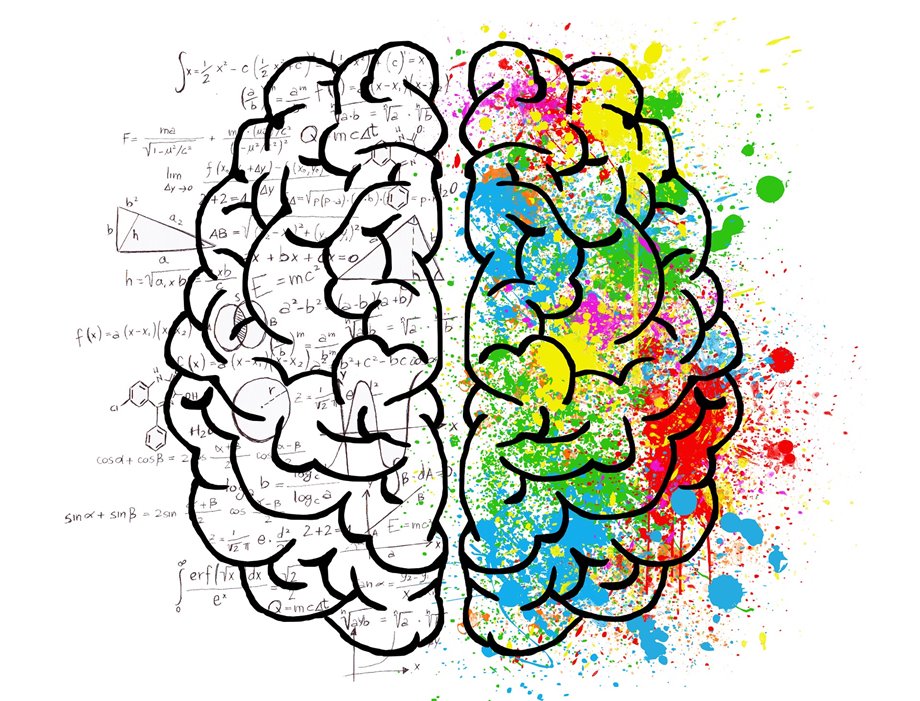
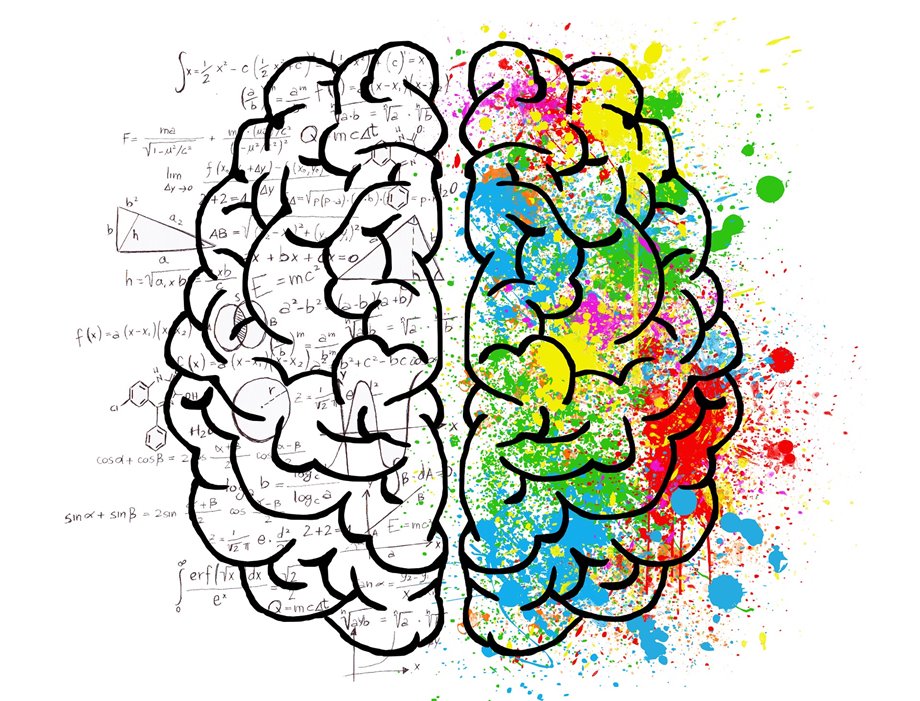
Researchers say that integrating AI and mathematical optimization tools will lead to a complete “paradigm shift” in business.
Fusing artificial intelligence with mathematical optimization will dramatically increase the “brainpower” for the task at hand, whether it’s optimizing flight patterns or bringing energy and food to underserved areas.
That’s the word from the academic researchers who are part of a new interdisciplinary institute that aims to integrate the two fields. The National AI Institute for Advances in Optimization (AI4OPT) is led by a multidisciplinary team from six U.S. universities, including computer science and civil, environmental, electrical, and computer engineering professors.
The combined methods will foster no less than a “paradigm shift” in optimization, said Pascal Van Hentenryck, professor of industrial and systems engineering at Georgia Tech and institute lead. According to Hentenryck, tackling problems at the scale and complexity faced by society today requires a fusion of optimization and machine learning, with the two technologies working hand-in-hand.
The two technologies use different techniques and algorithms and study different applications, Van Hentenryck said. The AI algorithms allow the software to learn over time in order to see patterns and expand on them, said Bistra Dilkina, its co-principal investigator.
“Although optimization largely works behind the scenes, it powers everything from supply chains and flight routes to package deliveries and even disaster response and organ allocation,” she said. “Without it, “the world would grind to a halt.”
Recommended for You: 6 AI and Machine Learning Tools for Engineers
The issues these technologies are asked to solve involve a vast number of options and variables. Through its machine-learning capabilities, AI can help make quick and efficient decisions, Van Hentenryck said.
“But as the world becomes increasingly interconnected, and also more uncertain, optimization problems have become daunting and incredibly challenging,” he said. In merging the two technologies, “the institute’s research will take a big step toward the science of good decision-making within large complex systems.”
The mission for AI4OPT is straightforward, if not simple: write programs that predict and quantify uncertainty, reason and optimize, and programs that continually learn, coordinate, collaborate, Dilkina said.
Harnessing both systems will help solve societal issues like unequal access to food and energy. Accordingly, one of the researcher’s first steps will be to write algorithms to get the most from controlled-environment agriculture systems (CEA).
Plants grown in small-scale plant beds will increase access to food for urban communities, Dilkina said. She’s a University of Southern California professor of computer science. The researchers are now designing testbeds that demonstrate the best use of domestic wastewater—rich in nutrients—within those systems. Within a CEA, plants are grown in soil rather than in water, inside a controlled environment like a greenhouse or a building.
The key for this project is mathematical optimization, which selects the best element—using principles set by researchers—from all available alternatives. The alternatives here include the many variables of introducing the wastewater such as when it’s applied, which nutrients it contains, and how often it’s applied, Dilkina said.
Listen to ASME TechCast: The AI Evolution of Generative Computer-Aided Design
The AI application determines over time the variables that work the best, which further narrows how the wastewater is used within the enclosed environment, Dilkina said. Mathematical optimization is used for far more than CEA, she adds. The team will put combined AI and machine learning to use in other “areas of significant societal impact,” Van Hentenryck said.
These include the use of massive, distributed renewable resources and energy demand response programs; green supply chains; and circuit design. At Berkeley, Alper Atamtürk said his team also has ambitious goals. Atamtürk is a professor of industrial engineering and the university’s lead for the institute.
His group is looking at how manufacturers could cut supply chain costs by scheduling and planning energy use and fuel consumption. It may seem small, but being able to adjust for energy use “can create major advances in areas of increasingly high demands such as same- or next-day shipping,” Atamtürk said.
Atamtürk echoes other researchers when he emphasizes how the institute “can lead to groundbreaking research, perhaps in ways we haven’t yet envisioned.”
As it turns out, changing the world for the better can involve a lot of math.
Jean Thilmany is a science and technology writer based in St. Paul, Minn.
That’s the word from the academic researchers who are part of a new interdisciplinary institute that aims to integrate the two fields. The National AI Institute for Advances in Optimization (AI4OPT) is led by a multidisciplinary team from six U.S. universities, including computer science and civil, environmental, electrical, and computer engineering professors.
The combined methods will foster no less than a “paradigm shift” in optimization, said Pascal Van Hentenryck, professor of industrial and systems engineering at Georgia Tech and institute lead. According to Hentenryck, tackling problems at the scale and complexity faced by society today requires a fusion of optimization and machine learning, with the two technologies working hand-in-hand.
The two technologies use different techniques and algorithms and study different applications, Van Hentenryck said. The AI algorithms allow the software to learn over time in order to see patterns and expand on them, said Bistra Dilkina, its co-principal investigator.
“Although optimization largely works behind the scenes, it powers everything from supply chains and flight routes to package deliveries and even disaster response and organ allocation,” she said. “Without it, “the world would grind to a halt.”
Recommended for You: 6 AI and Machine Learning Tools for Engineers
The issues these technologies are asked to solve involve a vast number of options and variables. Through its machine-learning capabilities, AI can help make quick and efficient decisions, Van Hentenryck said.
“But as the world becomes increasingly interconnected, and also more uncertain, optimization problems have become daunting and incredibly challenging,” he said. In merging the two technologies, “the institute’s research will take a big step toward the science of good decision-making within large complex systems.”
The mission for AI4OPT is straightforward, if not simple: write programs that predict and quantify uncertainty, reason and optimize, and programs that continually learn, coordinate, collaborate, Dilkina said.
Harnessing both systems will help solve societal issues like unequal access to food and energy. Accordingly, one of the researcher’s first steps will be to write algorithms to get the most from controlled-environment agriculture systems (CEA).
Plants grown in small-scale plant beds will increase access to food for urban communities, Dilkina said. She’s a University of Southern California professor of computer science. The researchers are now designing testbeds that demonstrate the best use of domestic wastewater—rich in nutrients—within those systems. Within a CEA, plants are grown in soil rather than in water, inside a controlled environment like a greenhouse or a building.
The key for this project is mathematical optimization, which selects the best element—using principles set by researchers—from all available alternatives. The alternatives here include the many variables of introducing the wastewater such as when it’s applied, which nutrients it contains, and how often it’s applied, Dilkina said.
Listen to ASME TechCast: The AI Evolution of Generative Computer-Aided Design
The AI application determines over time the variables that work the best, which further narrows how the wastewater is used within the enclosed environment, Dilkina said. Mathematical optimization is used for far more than CEA, she adds. The team will put combined AI and machine learning to use in other “areas of significant societal impact,” Van Hentenryck said.
These include the use of massive, distributed renewable resources and energy demand response programs; green supply chains; and circuit design. At Berkeley, Alper Atamtürk said his team also has ambitious goals. Atamtürk is a professor of industrial engineering and the university’s lead for the institute.
His group is looking at how manufacturers could cut supply chain costs by scheduling and planning energy use and fuel consumption. It may seem small, but being able to adjust for energy use “can create major advances in areas of increasingly high demands such as same- or next-day shipping,” Atamtürk said.
Atamtürk echoes other researchers when he emphasizes how the institute “can lead to groundbreaking research, perhaps in ways we haven’t yet envisioned.”
As it turns out, changing the world for the better can involve a lot of math.
Jean Thilmany is a science and technology writer based in St. Paul, Minn.