Digital Twins of Nuclear Power Plants
Digital Twins of Nuclear Power Plants
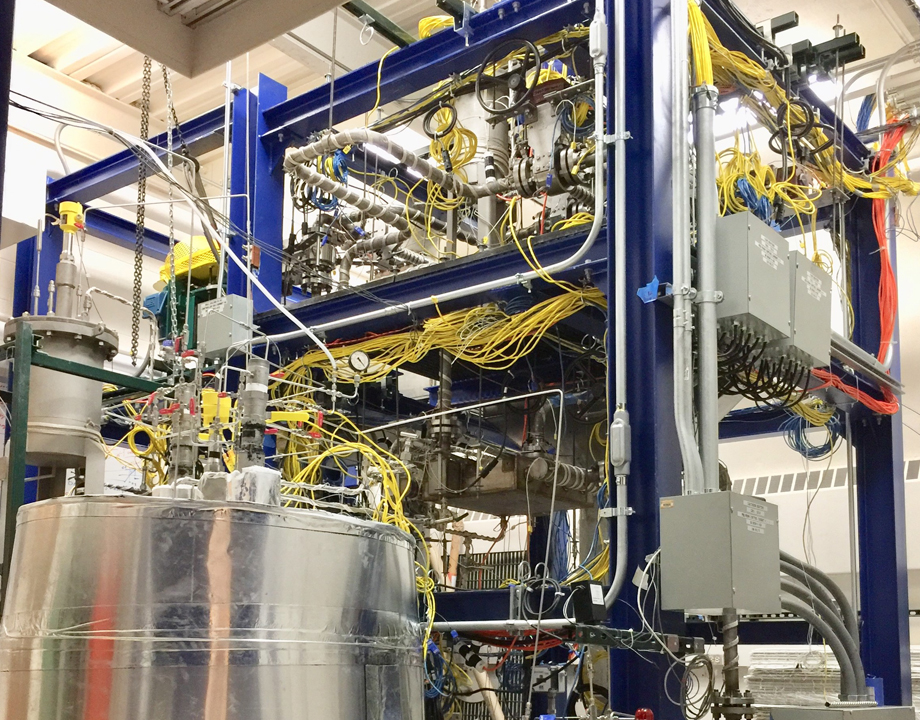
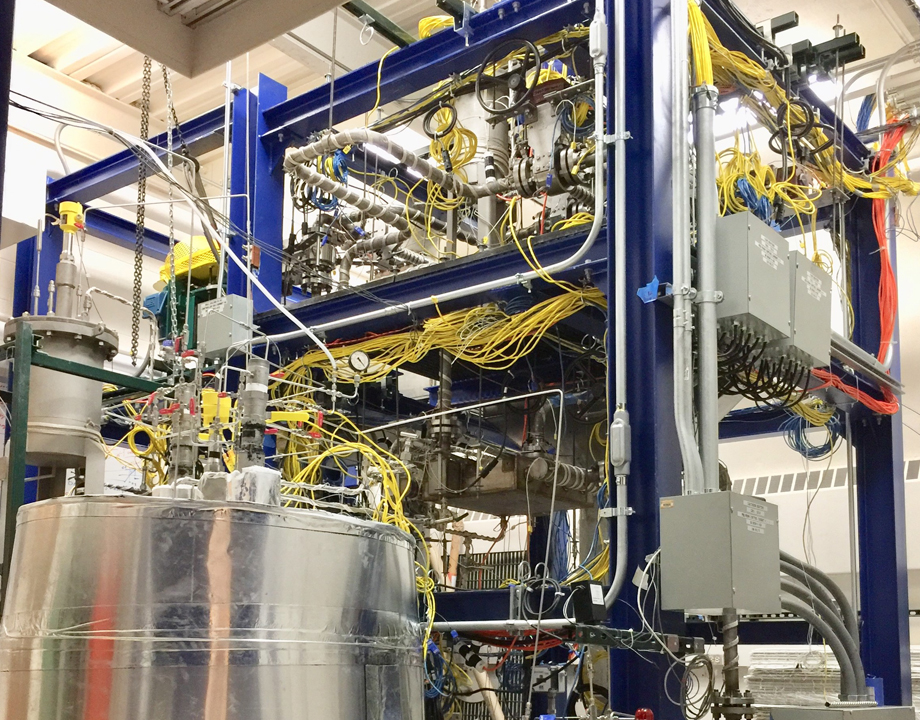
The experimental flow loop that will be used to emulate the cooling loop in a molten salt reactor. Photo: Xiaodong Sun, High-temperature Fluoride
A multidisciplinary team is developing digital twins of nuclear reactors with the help of a $5.2-million grant from the Department of Energy’s Advanced Research Projects Agency–Energy. The team is made of faculty at the University of Michigan, senior scientists at Idaho National Laboratory and Argonne National Laboratory, and industry partners at Kairos Power and Curtiss-Wright.
While machine learning algorithms will drive the digital twin, its predictions will be based on actual physics so the AI components don’t fall into an inscrutable black box, said principal investigator Annalisa Manera, a professor of nuclear engineering and radiological sciences at the University of Michigan in Ann Arbor. Having an ML-driven, physics-based model makes it more robust and reliable, Manera said.
The hope is that ML-driven predictive maintenance can shave some of the cost of constructing new nuclear plants. Using physics-based ML-driven models will help predict that a certain component will fail before it actually does. “This way we can achieve two things. First, we can avoid an unexpected outage. Second, you can start optimizing maintenance,” Manera said. Given that plant parts are often replaced on a predetermined schedule, using the digital twin could allow for more granularity in maintenance. Both results save costs.
A complex operating environment such as a nuclear power plant has different components, each with several interconnections. The ML-driven digital twin can help understand these interconnections and identify which component is actually failing. When you have a digital twin, Manera said, you can compare the data you’re measuring against what you’re expecting to measure and see how the two stack up. Deviations, if any, can be a sign of trouble. “The question is what’s the chicken and what’s the egg. And that’s where the digital twin can help,” she added.
Recommended for You: 7 Digital-Twin Applications for Manufacturing
The ML-enhanced digital twin can also support flexible operation of nuclear power plants. Whereas typically a nuclear plant would operate at maximum power constantly, what is known as base load, there is more demand for nuclear energy to be more responsive to market status.
“Where we have a lot of intermittent power from renewables, there’s a growing need for the nuclear power plant to start operating [in load-follow mode],” she said. This means that if there’s a lower request for nuclear power due to distributed energy sources and increased flow from solar or wind, then the plant should accordingly decrease production—and vice versa.
Manera said it’s challenging to find a common language for the multidisciplinary team to work together. The other challenge is training a machine learning model with limited amounts of data. The solution is two-pronged: Set the complex experimental facility at the university to induce artificial failure of components and generate additional data sets. Second, have very high-fidelity digital twins.
Listen to a Podcast: Leveraging Digital Twins for Aerospace and Defense
“These are accurate, but computationally expensive first-principled models that can help bridging the gap between the fast-running, ML-enhanced lowfidelity digital twins and the plant/experimental data needed for the AI training,” said Manera.
The digital twin is a central piece of the software being developed, which contains several other modules: maintenance predictive module, operation controller module, supervisor module. The final goal is to develop the complete software package that other vendors can use, after plugging their specific models/information.
The current one will be based on the Kairos Power fluoride-salt-cooled, high-temperature reactor, one of a number of new advanced plant designs being proposed. “Our idea behind this project is that we can support not only reactors that are already operating, but also reactors that are in the design phase because we can use a very high-resolution digital twin combined with experimental data,” she said.
The digital twin can help save money in the design process too. “The more you can do upfront with simulations to inform more focused experimental tests, the more time and money you can save,” Manera said.
Poornima Apte is an independent writer based in Walpole, Mass.
Register Today for ASME’s Digital Twin Summit
While machine learning algorithms will drive the digital twin, its predictions will be based on actual physics so the AI components don’t fall into an inscrutable black box, said principal investigator Annalisa Manera, a professor of nuclear engineering and radiological sciences at the University of Michigan in Ann Arbor. Having an ML-driven, physics-based model makes it more robust and reliable, Manera said.
The hope is that ML-driven predictive maintenance can shave some of the cost of constructing new nuclear plants. Using physics-based ML-driven models will help predict that a certain component will fail before it actually does. “This way we can achieve two things. First, we can avoid an unexpected outage. Second, you can start optimizing maintenance,” Manera said. Given that plant parts are often replaced on a predetermined schedule, using the digital twin could allow for more granularity in maintenance. Both results save costs.
Comparing Data
A complex operating environment such as a nuclear power plant has different components, each with several interconnections. The ML-driven digital twin can help understand these interconnections and identify which component is actually failing. When you have a digital twin, Manera said, you can compare the data you’re measuring against what you’re expecting to measure and see how the two stack up. Deviations, if any, can be a sign of trouble. “The question is what’s the chicken and what’s the egg. And that’s where the digital twin can help,” she added.
Recommended for You: 7 Digital-Twin Applications for Manufacturing
The ML-enhanced digital twin can also support flexible operation of nuclear power plants. Whereas typically a nuclear plant would operate at maximum power constantly, what is known as base load, there is more demand for nuclear energy to be more responsive to market status.
“Where we have a lot of intermittent power from renewables, there’s a growing need for the nuclear power plant to start operating [in load-follow mode],” she said. This means that if there’s a lower request for nuclear power due to distributed energy sources and increased flow from solar or wind, then the plant should accordingly decrease production—and vice versa.
Upfront Simulation
Manera said it’s challenging to find a common language for the multidisciplinary team to work together. The other challenge is training a machine learning model with limited amounts of data. The solution is two-pronged: Set the complex experimental facility at the university to induce artificial failure of components and generate additional data sets. Second, have very high-fidelity digital twins.
Listen to a Podcast: Leveraging Digital Twins for Aerospace and Defense
“These are accurate, but computationally expensive first-principled models that can help bridging the gap between the fast-running, ML-enhanced lowfidelity digital twins and the plant/experimental data needed for the AI training,” said Manera.
The digital twin is a central piece of the software being developed, which contains several other modules: maintenance predictive module, operation controller module, supervisor module. The final goal is to develop the complete software package that other vendors can use, after plugging their specific models/information.
The current one will be based on the Kairos Power fluoride-salt-cooled, high-temperature reactor, one of a number of new advanced plant designs being proposed. “Our idea behind this project is that we can support not only reactors that are already operating, but also reactors that are in the design phase because we can use a very high-resolution digital twin combined with experimental data,” she said.
The digital twin can help save money in the design process too. “The more you can do upfront with simulations to inform more focused experimental tests, the more time and money you can save,” Manera said.
Poornima Apte is an independent writer based in Walpole, Mass.
Register Today for ASME’s Digital Twin Summit