Bioengineering Blog: AI and Deep Learning Save Research Time During a Pandemic
Bioengineering Blog: AI and Deep Learning Save Research Time During a Pandemic
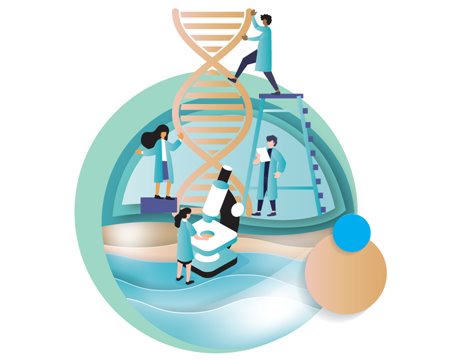
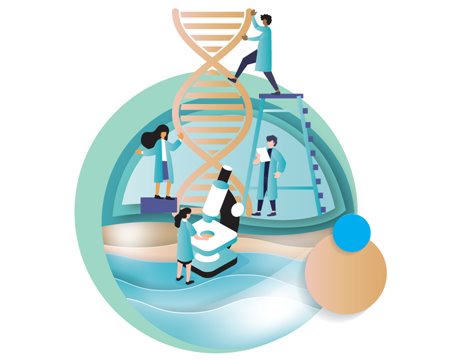
Many of the successful applications of simulation, machine learning, and additive manufacturing will be highlighted over the coming months as the world addresses the COVID-19 pandemic.
In bioengineering, the time it takes to develop and field a solution has always been a concern when the solution will improve or save the lives of patients. Within the news cycles of the developing COVID-19 pandemic there has been a focus on the time it takes to develop new vaccines and critical medical equipment. Many of the same technologies we have been talking about here in the ASME blog are now being harnessed to find solutions to the challenges presented by the pandemic.
Countless examples of computational modeling and simulation being used to project disease progression highlight the potential impact of proposed mitigation efforts, and even explain to the public how individual actions impact the disease spread. In an interview with National Public Radio, Caitlin Rivers, an infectious disease modeler with the Johns Hopkins Center for Health Security, said, “Modeling plays a really important roll in understanding how an outbreak is unfolding, where it might be going, and what we should be thinking through.”
Speed is of the essence for finding effective drugs and vaccines, so the computer systems at Oak Ridge National Laboratories have been put into service to screen a large number of molecules and identify promising compounds that may be effective in treating SARS-CoV-2 (the virus that causes COVID-19). Using the Summit supercomputer, researchers were able to screen 8,000 different compounds and identify 77 small-molecule drugs that may warrant further study, reported the lab. Quickly slimming down the testing field helps focus the limited laboratory testing in areas that have a higher potential success rate.
Related Bioengineering Blog: Supply Chains and the Health Crisis
As health systems are strained, time-intensive diagnostics that rely on specialized training will slow the identification of infected patients allowing the virus to spread even further before isolation. Being a worldwide pandemic, this problem is exacerbated for countries with more limited resources. In the hopes of reducing that load on healthcare workers, machine learning and AI systems are being trained to help diagnose COVID-19 patients by their CT scans or Chest Radiographic images (CXR). Early results are promising, but further development and testing is needed before a solution can be deployed around the world, according to Towards Data Science.
Additive manufacturing and 3D printing technologies are now being used to manage supply gaps during patient treatment. In Italy, where hospitals are overwhelmed and shortages of key equipment such as breathing machines are common, a hospital has been 3D printing replacement valves to connect patients to the equipment. Shortages that would have left equipment unused and patients untreated have been addressed with a stopgap measure of printing the parts locally.
Related Video: US Begins First Human Trial of Coronoavirus Vaccine
Many of the successful applications of simulation, machine learning, and additive manufacturing will be highlighted over the coming months as the world addresses the COVID-19 pandemic. The long-term advantage is to open the door to new approaches that will save development time and get safe and effective medical treatments to patients as quickly as possible, not only during global health emergencies, but also as a bioengineering approach for many problems going forward.
Arlen Ward, PhD, PE, is a Modeling and Simulation Principal for System Insight Engineering, a consultancy providing computational modeling and simulation services for medical device companies.
Opinions expressed are the author’s and do not necessarily reflect the views of ASME.
Countless examples of computational modeling and simulation being used to project disease progression highlight the potential impact of proposed mitigation efforts, and even explain to the public how individual actions impact the disease spread. In an interview with National Public Radio, Caitlin Rivers, an infectious disease modeler with the Johns Hopkins Center for Health Security, said, “Modeling plays a really important roll in understanding how an outbreak is unfolding, where it might be going, and what we should be thinking through.”
Speed is of the essence for finding effective drugs and vaccines, so the computer systems at Oak Ridge National Laboratories have been put into service to screen a large number of molecules and identify promising compounds that may be effective in treating SARS-CoV-2 (the virus that causes COVID-19). Using the Summit supercomputer, researchers were able to screen 8,000 different compounds and identify 77 small-molecule drugs that may warrant further study, reported the lab. Quickly slimming down the testing field helps focus the limited laboratory testing in areas that have a higher potential success rate.
Related Bioengineering Blog: Supply Chains and the Health Crisis
As health systems are strained, time-intensive diagnostics that rely on specialized training will slow the identification of infected patients allowing the virus to spread even further before isolation. Being a worldwide pandemic, this problem is exacerbated for countries with more limited resources. In the hopes of reducing that load on healthcare workers, machine learning and AI systems are being trained to help diagnose COVID-19 patients by their CT scans or Chest Radiographic images (CXR). Early results are promising, but further development and testing is needed before a solution can be deployed around the world, according to Towards Data Science.
Additive manufacturing and 3D printing technologies are now being used to manage supply gaps during patient treatment. In Italy, where hospitals are overwhelmed and shortages of key equipment such as breathing machines are common, a hospital has been 3D printing replacement valves to connect patients to the equipment. Shortages that would have left equipment unused and patients untreated have been addressed with a stopgap measure of printing the parts locally.
Related Video: US Begins First Human Trial of Coronoavirus Vaccine
Many of the successful applications of simulation, machine learning, and additive manufacturing will be highlighted over the coming months as the world addresses the COVID-19 pandemic. The long-term advantage is to open the door to new approaches that will save development time and get safe and effective medical treatments to patients as quickly as possible, not only during global health emergencies, but also as a bioengineering approach for many problems going forward.
Arlen Ward, PhD, PE, is a Modeling and Simulation Principal for System Insight Engineering, a consultancy providing computational modeling and simulation services for medical device companies.
Opinions expressed are the author’s and do not necessarily reflect the views of ASME.